Mapping the history of the Milky Way with Machine Learning
- Tiago Campante
- Mar 30
- 2 min read
The Milky Way is a complex tapestry of stars, shaped by billions of years of evolution, mergers, and dynamic interactions. Understanding its history requires identifying different stellar populations — groups of stars that share common origins and properties. Traditionally, astronomers have relied on chemical composition, motion, and age to classify these populations. However, because these characteristics often overlap, distinguishing one group from another remains a challenge.
In an article led by my grad student Andreas Neitzel, published last week in the peer-reviewed journal Astronomy & Astrophysics (link to the article here), we introduce an innovative approach to this problem by applying machine learning techniques — specifically, manifold learning — to analyze large datasets of stars. Manifold learning is an advanced method that can uncover hidden structures in high-dimensional data without relying on predefined assumptions about the number or nature of stellar populations. We use the Uniform Manifold Approximation and Projection (UMAP) algorithm, a state-of-the-art dimensionality reduction technique, to extract meaningful patterns from the data. By letting the data speak for itself, this approach potentially allows for a more nuanced and accurate classification of stars in the Milky Way.
To test this methodology, we used simulated stellar data (specifically, we have picked a Milky Way-like galaxy from the FIRE-2 cosmological zoom-in simulations) modeled after the observations collected by the Gaia mission. These simulated stellar data represent red-giant stars whose ages can be precisely measured using asteroseismology — the study of stellar oscillations. By applying UMAP, we reduced the complex five-dimensional input parameter space of stellar properties into a two-dimensional representation. This allowed us to visually and quantitatively assess how well our method distinguishes between different stellar populations.
Our results demonstrate that UMAP effectively identifies distinct groups of stars, revealing patterns that traditional techniques may overlook (see Fig. 1). This ability to disentangle overlapping stellar populations provides a more detailed understanding of the Galaxy’s evolutionary history. Furthermore, this method holds promise for refining the classification of stars in future observational surveys, particularly as new high-precision datasets become available.
Looking ahead, our work paves the way for more sophisticated applications of machine learning in astrophysics. As the field moves towards increasingly data-driven approaches, methodologies like UMAP will become essential for unlocking the full potential of astronomical surveys. By improving our ability to classify and analyze stellar populations, we take another step toward deciphering the Milky Way’s rich and complex past.
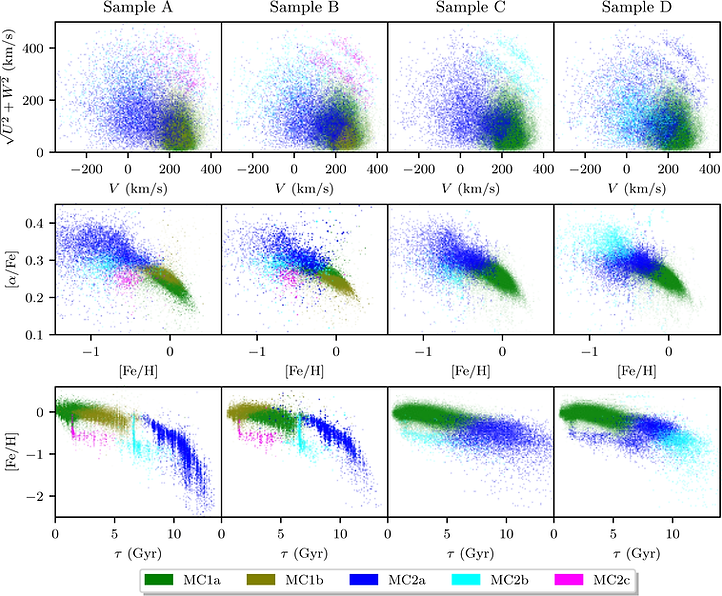
Comments